The Disparate Effects of AI on the Environment
News Mania Desk / Piyal Chatterjee / 16th April 2025
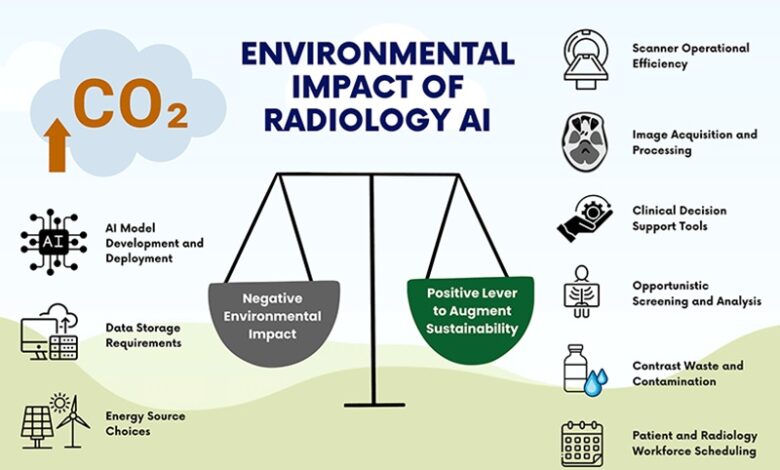
The implementation of artificial intelligence has been quickly increasing throughout all sectors of society, offering the possibility to tackle common global issues like climate change and drought management. However, beneath the enthusiasm for AI’s transformative possibilities lie ever-larger and more energy-demanding deep neural networks. The increasing demands of these intricate models are causing worries about AI’s effect on the environment.
Crucially, in addition to their worldwide climate influence, the environmental consequences of AI hold considerable importance at both local and regional scales. Although recent efforts offer hopeful advances for sustainable AI, they frequently focus on easily quantifiable environmental indicators like the overall carbon emissions and water usage. They fail to adequately address environmental equity — the necessity that AI’s environmental expenses are fairly shared among various regions and communities.
Even disregarding the environmental impact of chip production and supply chains, training one AI model, like a large language model, can use thousands of megawatt hours of electricity and release hundreds of tons of carbon emissions. This is approximately comparable to the yearly carbon output of hundreds of homes in the United States. Moreover, the training of AI models can result in the loss of a significant quantity of fresh water into the atmosphere due to data center cooling, possibly worsening the pressure on our already scarce freshwater supplies.
All these ecological effects are anticipated to rise significantly, with the worldwide AI energy needs estimated to grow exponentially to at least 10 times the existing level, surpassing the yearly electricity usage of a small nation such as Belgium by 2026. In the United States, the swiftly increasing demand for AI is expected to propel data center energy consumption to approximately 6% of the country’s overall electricity usage by 2026.
The production of electricity, especially via fossil fuel burning, leads to local air contamination, thermal pollution in aquatic environments, and the creation of solid waste, including hazardous substances. Increased carbon emissions in an area incur specific social costs, which may result in elevated levels of ozone, particulate matter, and early deaths. Moreover, the pressure on local freshwater supplies from the significant water use linked to AI, both for onsite server cooling and indirectly for offsite power generation, can exacerbate extended droughts in water-scarce areas such as Arizona and Chile.
During a time of increased awareness about environmental issues, numerous complex initiatives have been emerging, focused on promoting AI’s sustainability and securing its beneficial role in addressing climate change. Improvements in power and cooling systems for data centers have significantly lowered the previously high energy costs of AI computation, demonstrated by the reduction in power usage effectiveness (PUE) from 2.0 to 1.1 or even less in state-of-the-art data center environments.
Additional significant innovations encompass the development of effective AI model architectures, optimization methods that speed up AI training and inference, strategies such as weight pruning and quantization for minimizing model sizes, and the advancement of energy-efficient GPUs and accelerators.
At the system level, comprehensive management of both computing and non-computing resources is crucial for creating sustainable AI systems. For example, geographical load balancing, a proven method, can dynamically match energy demand with current grid operating conditions and carbon intensities across a network of decentralized data centers. Its success in reducing environmental impact has been shown in practical systems, including Google’s carbon-smart computing platform.
Regrettably, a growing gap persists in the ways various regions and communities experience the environmental consequences of AI. In numerous instances, negative environmental effects of AI disproportionately affect communities and areas that are especially susceptible to the ensuing ecological damage. For example, in 2022, Google powered its data center in Finland with 97% carbon-free energy; this figure falls to 4–18% for its centers in Asia. This emphasizes a notable imbalance in the regional use of fossil fuels and the generation of air pollution. Likewise, the water usage rate for cooling data centers can be significantly elevated in drought-affected areas like Arizona because of their warmer climates.
Furthermore, current methods for implementing and overseeing AI computing frequently worsen environmental inequality, a situation that is intensified by ongoing socioeconomic differences across areas. For example, geographical load balancing that focuses on minimizing total energy costs or carbon emissions might unintentionally raise the water footprint of data centers in regions facing water stress, which would further pressure local freshwater supplies. It might also unevenly increase grid congestion and elevate locational marginal prices for electricity, which could result in higher utility rates and unfairly impact local residents with increased energy expenses.
Indeed, ensuring consistent environmental impact for AI data centers in all geographical areas is difficult, since certain regions naturally encounter more environmental disadvantages than others.
Nonetheless, the capability to dynamically implement and oversee AI computing across a network of geographically spread data centers presents significant chances to address AI’s environmental disparities by emphasizing underprivileged areas and fairly allocating the total adverse environmental effects. Although redirecting vehicle traffic on a freeway to tackle the related air pollution poses logistical challenges, implementing geographical load balancing to spatially shift “AI traffic,” such as AI training jobs and specific inference requests, is straightforward and can be done right away. This method allows for the real-time redistribution of AI computing among geographically diverse data centers, taking into account immediate local elements like the share of fossil fuel energy and water efficiency. Essentially, it holds true whether a business manages its own geographically dispersed data center systems or utilizes cloud-based AI solutions. For example, a large tech firm can effortlessly transfer the majority of its computing operations to different data centers without affecting its services, while a small enterprise can adaptively and flexibly relocate its computing requirements among various cloud regions.
By evenly distributing AI computing among various data centers, we can efficiently reallocate AI’s environmental costs by region, promoting a more balanced result. The main innovation focuses on prioritizing areas experiencing the most significant negative environmental effects, like increased air pollution and diminishing freshwater resources. This is achieved by dynamically allocating a greater importance weight to regions that are otherwise excessively burdened while optimizing geographical load balancing choices in real time.